|
         
Tutorial Sessions
All
tutorials are free to registered conference attendees of
all conferences held at WOLDCOMP'10. Those who are
interested in attending one or more of the tutorials are
to sign up on site at the conference registration desk
in Las Vegas.
A
complete & current list of WORLDCOMP Tutorials
can be found
here.
In addition to
tutorials at other conferences, DMIN'10 aims at
providing a set of tutorials dedicated to Data Mining
topics. The 2007 key tutorial was given
by Prof. Eamonn
Keogh on Time Series Clustering. The 2008 key tutorial
was presented by Mikhail Golovnya (Senior Scientist,
Salford Systems, USA) on Advanced Data Mining
Methodologies.
DMIN'09 provided four
tutorials presented by Prof. Nitesh V. Chawla on Data Mining with
Sensitivity to Rare Events and Class Imbalance,
Prof. Asim Roy on Autonomous Machine
Learning, Dan Steinberg (CEO of Salford Systems)
on Advanced
Data Mining Methodologies, and Peter Geczy on Emerging Human-Web
Interaction Research.
DMIN'10
will host the following tutorials:
Tutorial A |
Speaker: |
Prof. Vladimir
Cherkassky
Fellow of IEEE;
ECE Department, University of Minnesota,
Minneapolis, MN, USA;
Former Director, NATO Advanced Study Institute
(ASI);
www.ece.umn.edu/users/cherkass/predictive_learning
Served on the editorial boards of IEEE
Transactions on Neural Networks,
the Neural Networks Journal, the Natural
Computing Journal and the Neural Processing
Letters |
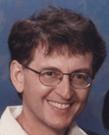
|
Topic: |
Advanced
Methodologies for Learning with Sparse Data |
Webpage |
http://www.ece.umn.edu/users/cherkass/predictive_learning/ |
Date & Time |
July 13, 2010
(6:00pm - 9:30pm) |
Location |
Ballroom 5 |
Description |
OVERVIEW:
The field of Predictive
Learning is concerned with estimating ‘good’
predictive models from available data. Such
problems can be usually stated in the framework
of inductive learning, where the goal is
to come up with a good predictive model from
known observations (or training data samples).
In recent years, there has been a growing
interest in applying learning methods to sparse
high-dimensional data (i.e., in genomics,
medical imaging, object recognition, etc.). In
such applications, many successful approaches
represent minor modifications of existing
inductive learning methods (such as neural
networks, support vector machines, discriminant
analysis etc.) combined with clever
preprocessing and feature extraction. At the
same time, in the statistical learning
community, there is a trend towards development
and better understanding of new non-standard
and non-inductive learning settings.
Examples include (a) several powerful learning
formulations developed in VC-theory:
transduction, learning through contradictions,
SVM+ (Vapnik, 1998, 2006); and (b) non-standard
settings proposed in machine learning community,
such as Multi-Task Learning (Ben-David et al,
2002), Semi-Supervised Learning (Chapelle et al,
2006) etc.. These new learning formulations are
motivated by practical needs (to improve
generalization for learning with sparse
high-dimensional data). This tutorial will
present an overview of recent novel learning
formulations, investigate possible connections
between these formulations, and discuss
application examples illustrating advantages of
using these approaches for sparse
high-dimensional data. The presentation will be
based, to a large extent, on the conceptual
framework developed by Vapnik [1998, 2006].
CONTENT:
This tutorial will cover
three major parts. The first part will
present VC-theoretical framework for predictive
learning and discuss standard inductive learning
setting, in order to motivate alternative
approaches. Second part presents several
non-standard learning formulations such as
transduction, learning through contradictions,
learning with hidden information and multi-task
learning. In the third part, we discuss
practical issues and difficulties arising in
application of these advanced learning
techniques. Throughout this tutorial, many
important points will be illustrated by
empirical comparisons and related to practical
applications (mainly, biomedical applications).
TUTORIAL DURATION:
2.5 hours
INTENDED AUDIENCE:
Researchers and
practitioners interested in understanding
advanced learning methodologies, and their
applications. This tutorial is also helpful for
developing improved understanding of the
methodological issues for learning with
high-dimensional data.
References
S.
Ben-David, J. Gehrke and R. Schuller, A
theoretical framework for learning form a pool
of disparate data sources. ACM KDD, 2002.
O.
Chapelle, B. Schölkopf and A. Zien, Eds.,
Semi-Supervised Learning, MIT Press, 2006
Cherkassky, V. and Y. Ma,
Data complexity, margin-based learning and
Popper’s philosophy of inductive learning, in
Data Complexity in Pattern Recognition, M.
Basu and T. Ho , Eds, Springer, 2006
Cherkassky, V. and F. Mulier,
Learning from Data, second edition,
Wiley, 2007
Cherkassky, Cai, F., and L. Liang, Predictive
learning with sparse heterogeneous data, Proc
IJCNN 2009
Vapnik, V., Statistical
Learning Theory, Wiley, 1998
Vapnik, V., Empirical
Inference Science: Afterword of 2006, Springer
2006
|
Short Bio |
Vladimir Cherkassky is Professor of Electrical
and Computer Engineering at the University of
Minnesota. He received Ph.D. in Electrical
Engineering from University of Texas at Austin
in 1985. His current research is on methods for
predictive learning from data, and he has
co-authored a monograph Learning From Data
published by Wiley in 1998. Prof. Cherkassky has
served on the Governing Board of INNS. He has
served on editorial boards of IEEE
Transactions on Neural Networks, the
Neural Networks Journal, the Natural
Computing Journal and the Neural
Processing Letters. He served on the program
committee of major international conferences on
Artificial Neural Networks. He was Director of
NATO Advanced Study Institute (ASI) From
Statistics to Neural Networks: Theory and
Pattern Recognition Applications held in
France, in 1993. He presented numerous tutorials
on neural network and statistical methods for
learning from data. In 2007, he became Fellow of
IEEE, for ‘contributions and leadership in
statistical learning and neural network
research’. |
Tutorial B |
Speaker: |
Dr. Peter Geczy |
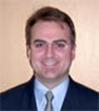
|
Topic: |
Web Mining:
Opportunities and Challenges |
Date & Time |
July 12, 2010
(6:50pm - 8:50pm) |
Location |
Ballroom 6 |
Description |
ABSTRACT:
Development of world wide web
has been influencing various domains of
commerce, government, and academia. Its
fast-paced growth and widespread adoption
inherently present numerous opportunities and
challenges. World wide web incorporates a broad
range of data available for exploration. Data is
significantly diverse, voluminous, and exhibits
dynamics reflecting its evolution. Researchers
and practitioners have been mining web data for
several decades, yet there is a plenty of more
to be done. We will briefly survey the status
quo, highlight selected approaches, and expose
possible promising directions in web mining.
OBJECTIVE:
The objective of this
tutorial is to provide concise overview of the
present state of issues, inherent difficulties,
contemporary approaches, and potential future
opportunities. Exposé of the state-of-the-art in
web mining should prove beneficial to a wide
spectrum of individuals researching, studying
and/or utilizing web mining techniques for both
academic and commercial purposes.
TUTORIAL DURATION:
approx. 2 hours
INTENDED AUDIENCE:
The tutorial aims to approach
a broad audience including, but not limited to:
- Students and
Educators
- Academics and Researchers
- Practitioners and Managers
The
presentation shall be in an accessible and
intuitive manner without extensive technical
details.
|
Short Bio |
Dr. Peter Geczy is a senior
scientist at The National Institute of Advanced
Industrial Science and Technology (AIST). He
also held positions at The Institute of Physical
and Chemical Research (RIKEN) and The Research
Center for Future Technology. His
interdisciplinary scientific interests encompass
domains of data and web mining, human
interactions and behavior in digital
environments, information systems, knowledge
management and engineering, artificial
intelligence, and machine learning. His recent
research focus also extends to the spheres of
service science, engineering, management, and
computing. He received several awards in
recognition of his accomplishments. Dr. Geczy
has been serving on various professional
committees, editorial boards, and has been a
distinguished speaker in academia and industry. |
Keynotes
Keynote |
Speaker: |
Prof. Vladimir
Cherkassky
Fellow of IEEE;
ECE Department, University of Minnesota,
Minneapolis, MN, USA;
Former Director, NATO Advanced Study Institute
(ASI);
www.ece.umn.edu/users/cherkass/predictive_learning
Served on the editorial boards of IEEE
Transactions on Neural Networks,
the Neural Networks Journal, the Natural
Computing Journal and the Neural Processing
Letters |
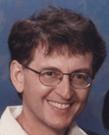
|
Topic: |
Predictive Data
Modeling and the Nature of Scientific Discovery |
Webpage |
www.ece.umn.edu/users/cherkass/predictive_learning |
Date & Time |
July 12, 2010
(06:00pm - 06:50pm) |
Location |
Ballroom 6 |
Description |
Abstract
Scientific
discovery involves interaction between two major
components:
-
facts, or observations of the Real World (or
Nature);
-
Scientific theories (models), i.e. mental
constructs, explaining this observed data.
In
classical science, the primary role belongs to a
well-defined scientific hypothesis which drives
data collection and generation. So experimental
data is simply used to confirm or refute a
scientific theory. In the late 20-th century,
the balance between facts and models in
scientific research has totally shifted, due to
a growing use of digital technology for data
collection and recording. Nowadays, there is an
abundance of available data describing physical,
biological and social systems. Several new
technologies, such as machine learning and data
mining, hold promise of â 'discovering' new
knowledge hidden in a sea of data. Much of
recent research in life sciences is data-driven,
i.e. when researchers try to establish 'associations'
between certain genetic variables and a disease.
This is completely different from the classical
approach to scientific discovery. Whereas many
machine learning and statistical methods can
easily detect correlations present in empirical
data, it is not clear whether such dependencies
constitute new biological knowledge. This is
known as the problem of demarcation in the
philosophy of science, i.e. differentiating
between true scientific theories and
metaphysical theories (beliefs).
Knowledge that can be extracted from empirical
data is statistical in nature, as opposed to
deterministic first-principle knowledge in
classical science. Modern science is mainly
about such an empirical knowledge, yet there
seems to be no clear demarcation between true
empirical knowledge and beliefs (supported by
empirical data). My talk will discuss
methodological issues important for predictive
data modeling, i.e.,
-
first-principle knowledge, empirical knowledge
and beliefs;
-
understanding of uncertainty and risk,
-
predictive data modeling,
-
interpretation of predictive models.
These methodological issues are closely related
to philosophical ideas, dating back to Plato and
Aristotle. The main points will be illustrated
by specific examples from an on-going project on
prediction of transplant-related mortality for
bone-and-marrow transplant patients, in
collaboration with the University of Minnesota
Medical School and the Mayo Clinic.
|
Short Bio |
Vladimir Cherkassky is Professor of Electrical
and Computer Engineering at the University of
Minnesota. He received Ph.D. in Electrical
Engineering from University of Texas at Austin
in 1985. His current research is on methods for
predictive learning from data, and he has
co-authored a monograph Learning From Data
published by Wiley in 1998. Prof. Cherkassky has
served on the Governing Board of INNS. He has
served on editorial boards of IEEE
Transactions on Neural Networks, the
Neural Networks Journal, the Natural
Computing Journal and the Neural
Processing Letters. He served on the program
committee of major international conferences on
Artificial Neural Networks. He was Director of
NATO Advanced Study Institute (ASI) From
Statistics to Neural Networks: Theory and
Pattern Recognition Applications held in
France, in 1993. He presented numerous tutorials
on neural network and statistical methods for
learning from data. In 2007, he became Fellow of
IEEE, for ‘contributions and leadership in
statistical learning and neural network
research’. |
|
   
|